Welcome!
We’re back once again with another packed edition of our Threat Modeling Insider! This month’s edition features a guest article by Jonathan Marcil, Application Security Specialist, on learning threats in a post ChatGPT world. Our curated content features a recording of the Open Security Summit on AI-Driven Threat Modeling with STRIDE GPT, and we provide a NIST Trustworthy and Responsible AI.
But that’s not all of course, let’s take a look at what else we have in store for this month’s edition:
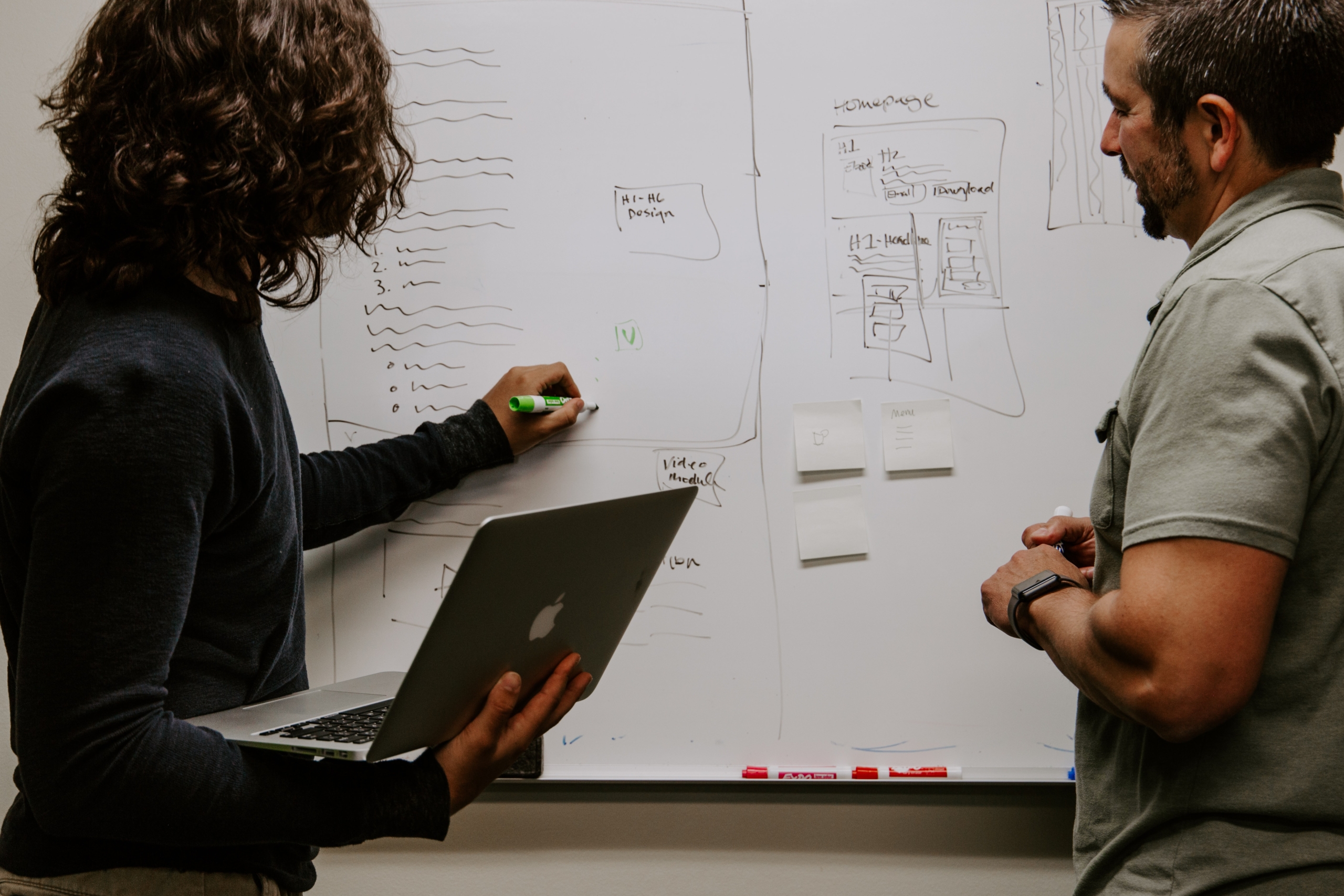